近年来,将变分同化和集合卡尔曼滤波同化相结合的集合-变分混合同化方法受到了广泛关注,该方法既保留了变分同化方法成熟有效的多源观测协调同化优势,又吸收了集合卡尔曼滤波同化的背景误差协方差可以随天气形势演变的优点。但是混合同化方法在每个同化时次仍需要一定的集合预报结果作为计算样本,这对于区域高分辨率实时同化预报而言,依然存在不小的计算压力,影响了其实际应用。
针对这一问题,陈耀登教授带领研究生开展了一系列攻关,提出了基于历史预报样本优选的集合-变分混合同化新方案。一系列单点观测理想试验和批量循环同化试验表明,该方案在不增加额外计算量的情况下,通过历史预报误差集合协方差的引入,能够给同化系统引入各向异性和一定“流依赖”特征的背景场误差协方差信息(图1),其预报效果明显优于类似计算量的三维变分方案(图2)。
由于历史预报样本具有一定的气候态特征,不能很好地表示“依流而变”的真实大气状态。为更好发挥历史预报样本节约计算量的优点,通过进一步对历史预报样本进行优选,从历史预报中自动地优选出与当前同化时刻天气形势相近的样本,将该样本作为混合同化中的集合预报样本。为期三周的批量连续循环同化及预报试验结果表明,本方案效果接近等集合量的常规混合同化方案,优于小样本的常规混合同化方案(图3)。
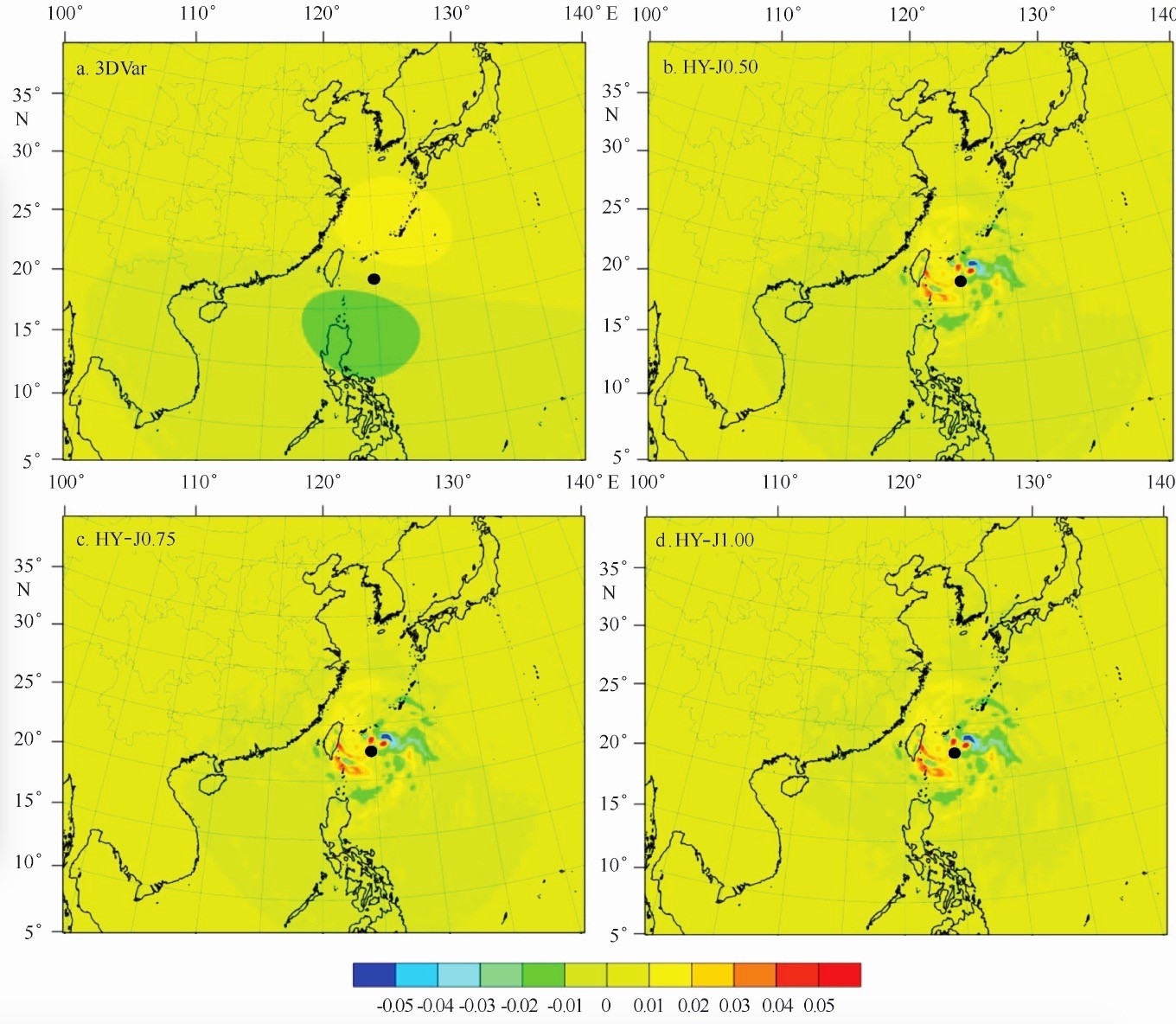
Fig.1. The temperature increments at the 10th level as a result of assimilating a single u-component of wind observation, and the innovation is 1.0 m/s
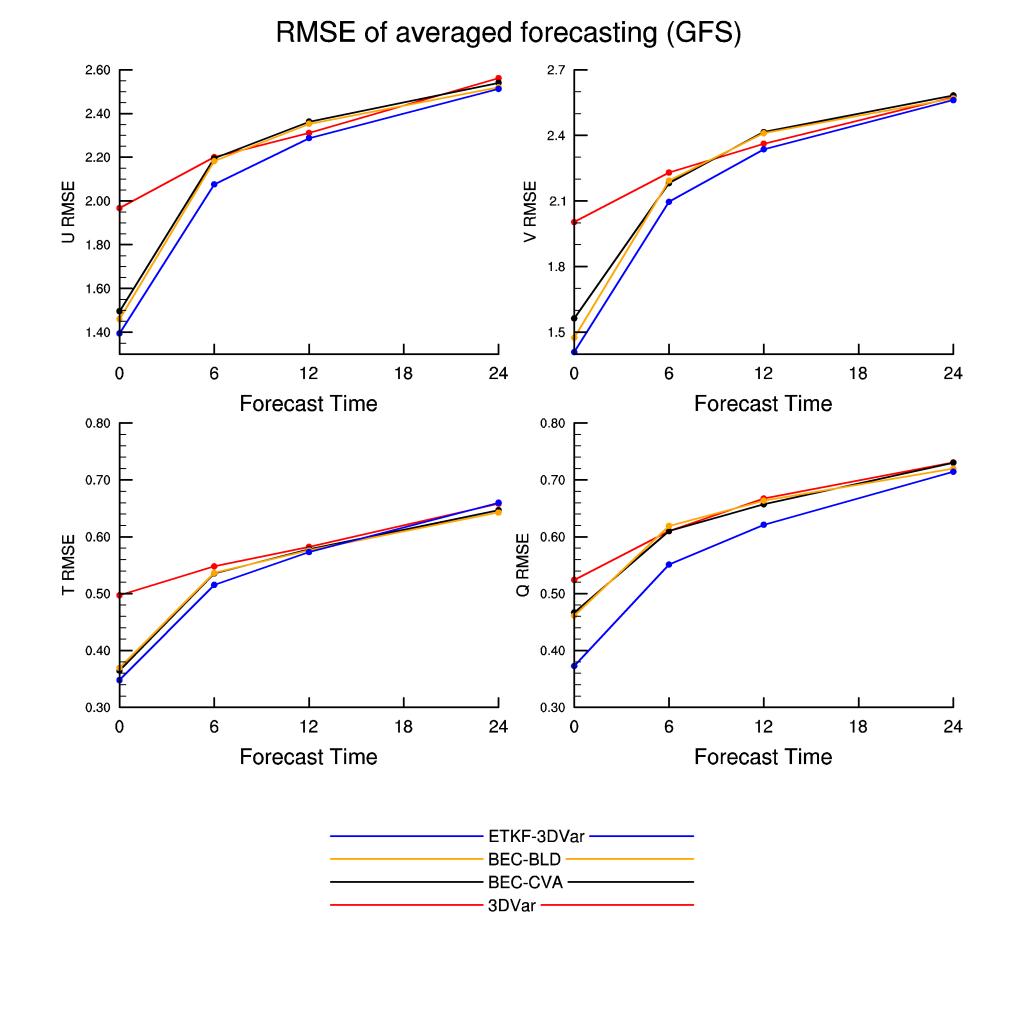
Fig.2. Vertical RMSE profiles of averaged analysis against GFS analyses. Red line denotes 3DVar, black line denotes BEC-CVA, orange line denotes BEC-BLD, blue line denotes ETKF-3DVar. Error bars show the confidence interval of the mean RMSE for that level (95% confidence limit).
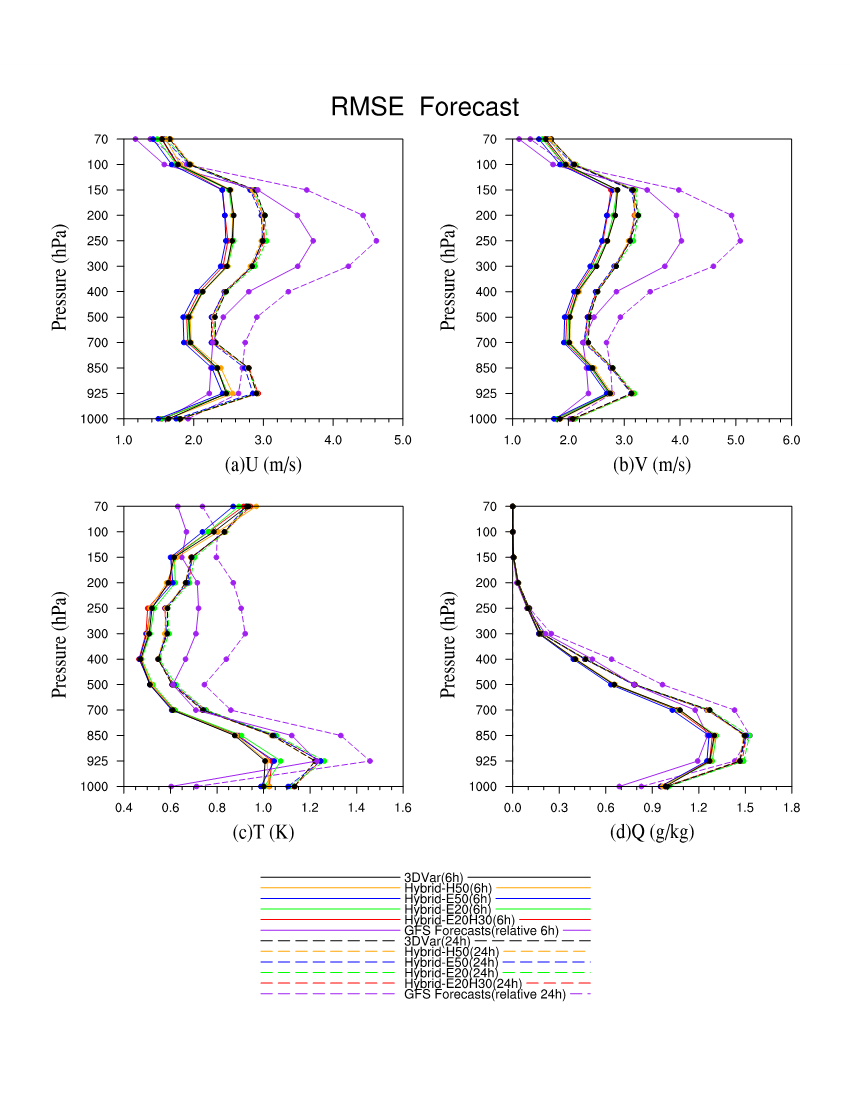
Fig. 3. Vertical profiles of the averaged RMSEs of 6 h forecast (solid line) and 24 h forecast (dotted line) RMSEs of (a) U (m s-1), (b) V (m s-1), (c) T (K) and (d) Q (g/kg) against the GFS analyses.
论文发表情况:
Yaodeng Chen*, Guo Shan, Deming Meng, et al. 2020. The impact of optimal selected historical forecasting samples on hybrid ensemble-variational data assimilation, Atmospheric Research, https://doi.org/10.1016/j.atmosres.2020.104980
Yaodeng Chen*, Jia Wang, Yufang Gao, et al. 2018. Refinement of the Use of Inhomogeneous Background Error Covariance Estimated from Historical Forecast Error Samples and its Impact on Short-term Regional Numerical Weather prediction. Journal of the Meteorological Society of Japan, 96(5): 429-446. https://doi.org/10.2151/jmsj.2018-048
陈耀登*,陈晓梦,闵锦忠等.各向异性背景场误差协方差的构建及在“凡亚比”台风的应用[J]. 海洋学报,2016,38(9):32-45.