诸多研究表明数值模式对云雨区和晴空区的模拟能力不同,云雨区和晴空区背景场误差存在一定的差异:有云和降水的区域背景场误差往往更大,水平长度尺度更小。因此,合理刻画云雨区水凝物背景场误差协方差有助于更好地同化云雨区观测资料,从而有效云雨区初始场,进而提升降雨预报准确度。
近日,我院陈耀登教授和孟德明博士开展了基于水凝物控制变量的云依赖同化研究。该工作在陈耀登教授团队前期基于水凝物控制变量的卫星云观测同化基础上,进一步提出了“云依赖”同化方案,并在变分框架下实现了各变量的云雨天气依赖分析(图1)。结果表明,“云依赖”同化技术可以合理地表征晴空区和云雨区不同的背景场误差协方差,从而实现卫星云观测云雨区和晴空区自适应分区同化(图2)。连续三周的批量对比试验表明,采用“云依赖”同化方案有效改善了云雨区水汽、风场、以及水凝物的分析和预报,进而改善了降雨尤其是暴雨的预报技巧(图3)。
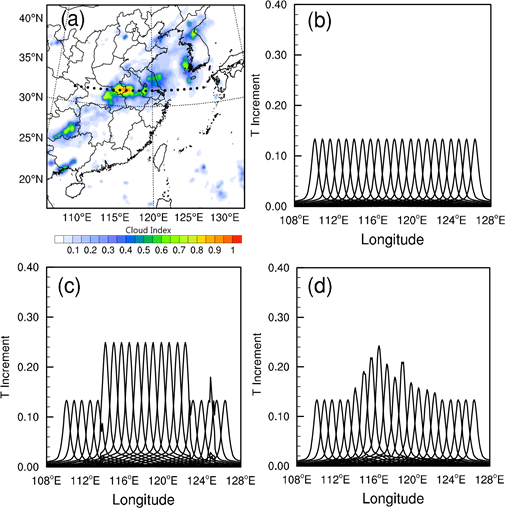
Figure 1. (a) The cloud index distribution (shaded) calculated from Cloud Water Path at 0000 UTC on 1 July 2016, and the distribution of the pseudo-observation point locations (black dots) for single observation tests. Each pseudo-observation point is located at 32°N, with a 1.5° longitude interval. The temperature increments (unit: K) along the line of latitude (32°N) that go through each single observation point at the 10th level of (b) the No_Adjust experiment, (c) the Adjust_no_Blur experiment, and (d) the Adjust_Blur experiment.
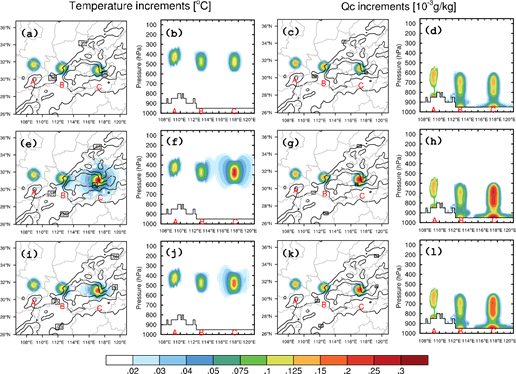
Figure 2. (a, e, i) The temperature increments (Shaded; unit: K) at the 10th level as a result of assimilating three pseudo temperature observations locating at A (clear sky area), B (edge of cloudy area), and C (cloudy area), respectively. Contours indicate the CI. The vertical cross sections of the temperature increment (b, f, j) is along 32°N. Figures (c, g, k) and (d, h, l) are the same as Figures (a, e, i) and (b, f, j), respectively, but for Qc increments. The results are from (a, b, c, d) the No_Adjust experiment, (e, f, g, h) the Adjust_no_Blur experiment, and (i, j, k, l) the Adjust_Blur experiment.
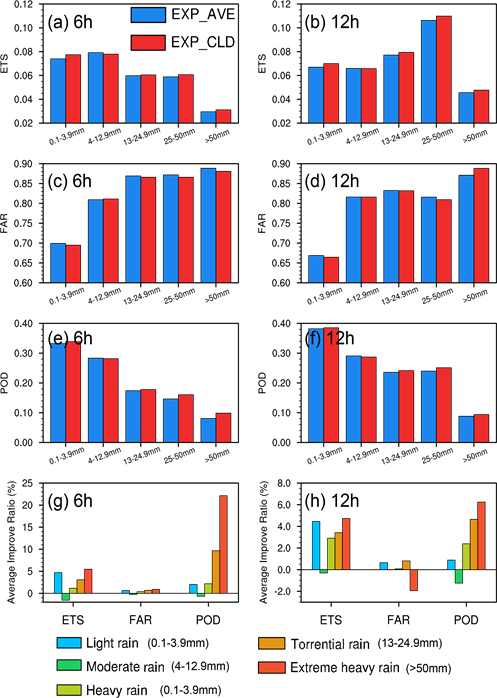
Figure 3. Rainfall forecast scores for (a, c, e) 6-h and (b, d, f) 12-h accumulated rainfall according to operational precipitation standard thresholds for (a, b) Equitable Threat Score (ETS), (c, d) False Alarm Ratio (FAR) and (e, f) Probability of Detection (POD). Average improvements in the percentage of the EXP_CLD experiment compared to the EXP_AVE experiment in different standard thresholds for (g) 6-h and (h) 12-h accumulated rainfall.
论文信息:
Meng, D., Chen, Y.*, Li, J., Wang, H., Wang, Y., & Sun, T. (2021). Cloud-dependent piecewise assimilation based on a hydrometeor-included background error covariance and its impact on regional Numerical Weather Prediction. Monthly Weather Review. https://doi.org/10.1175/MWR-D-20-0419.1